In the last couple of decades, road vehicles have increasingly been developed to include smart driving assist features to help prevent driver fatigue and help to avoid accidents with other vehicles and static objects – these are known as advanced driver assistance systems (ADAS).
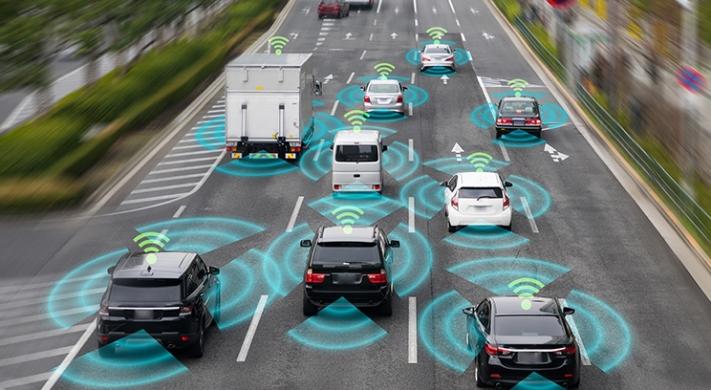
The relative simplicity of ADAS testing
Track testing of these ADAS features has matured and standardised such as with test definitions from Euro NCAP, NHTSA, and others. Using mobile platforms such as the Guided Soft Target (GST), LaunchPad, Soft Pedestrian Target (SPT), or real vehicles driven by AB Dynamics’ driving robots, these tests can be synchronised perfectly around a vehicle under test (VUT) using our patented Synchro software.
These innovations have allowed ADAS tests to be performed precisely, efficiently, and safely. Track testing an ADAS feature using one of our guided platforms has remained manageable since there is only one VUT and one other test object to consider - there are relatively few permutations to deal with in terms of the relative trajectories between the VUT and platform. For example, an automatic emergency braking system only needs to consider the relative speed, distance and time-to-collision of the car directly in front.
To an autonomous track testing future
The ultimate evolution of driving assistance features is to allow a vehicle to autonomously drive itself on public roads whilst not being a hazard to other road users – so-called autonomous vehicles. Additionally, both autonomous and human-driven vehicles will be connected in the next few years using Vehicle-to-Everything (V2X) technology. Vehicles will communicate with each other and to roadside infrastructure, such as traffic lights, to warn of hazards and dangers in the road ahead. These new complex technologies also require testing to ensure safe and reliable operation.
So how do we test these new systems? As we see it, autonomous vehicle testing has so far branched into four main categories, each with benefits and drawbacks:
- Simulation Data Farming is where many computer servers running software such as the rFpro Data Farming solution provide a simulated environment of scenery, moving objects, vehicle dynamics and sensor output to virtually test autonomous vehicle driving software. This is a software-only solution that can run faster or slower than real-time, and where an actual vehicle and sensor hardware are not required. This is used as an algorithm validation tool, but also to train the vehicle’s driving software by highlighting correct and unsafe behaviour, in a process known as supervised learning. This solution can significantly increase the volume of testing by several orders of magnitude but requires an accurately modelled world.
- With Vehicle-in-the-loop (VIL) testing, an autonomous vehicle is configured by engineers to operate in a lab, either when stationary or when driving on a dyno rig. Sensors that are built into vehicle view a simulated world of challenging driving scenarios - a blend and hardware and simulation. For example, the camera system on the autonomous vehicle would view 360° simulated imagery, or where synthetic GPS data is broadcast into the vehicle's antenna. VIL testing adds an element of realism since actual hardware used, whilst also making the test set-up and configuration much easier for the test engineers. But not every sensor hardware type can be used effectively in this way; and it does not provide all of the systemic subtleties of the real world, such as how a vehicle driving over a pothole causes extra motion blur on the on a camera sensor.
- Real-World Fleet Testing involves autonomous vehicles that are permitted to drive in real-world traffic under the supervision of a safety driver who is there prevent accidents if the driving software fails. Mistakes and good driving behaviour data are recorded, which can help to provide long-term statistics about performance, such as the mean time between failure (MTBF) - autonomous vehicle manufactures tend to talk about ‘disengagement rates’ (miles between incidents where human driver is forced to take back control). It also helps to train the vehicle’s driving software by feeding driving data back into the autonomous vehicle software using a supervised learning technique or to allow software engineers to diagnose and fix problems in the software. The randomness of the real world, however, makes it nearly impossible to check if a correction to the autonomous vehicle driving software has worked as intended as that exact situation may never be encountered again.
- With Track Testing, an autonomous vehicle is configured to drive on a test track along with a combination of soft guided robot targets, dynamically controlled roadside infrastructure and static road furniture. The aim is to make the scene as realistic as possible to what an autonomous vehicle will experience in the real-world – yet in a highly controlled and safe environment. This setup provides precise and realistic test scenarios but is time-consuming in comparison to simulation. These tests can be repeated and adjusted by the engineers if necessary to allow fine-tuning of software algorithms or provide validation with confidence.
Track testing is the only reliable way to test the most dangerous scenarios
With track testing of autonomous vehicles, however, the sheer number of permutations, variations in the scenario, and increase in road actors may seem like an impossible task – there are a near-infinite number of possibilities to test!
Whilst a large proportion of the testing can be carried out in simulation, the most hazardous or challenging scenarios will likely require track testing – and even with this constraint, the task remains daunting. Yet, there is no safer and more reliable way to test the complete autonomous vehicle system with close-in dangerous scenarios than by performing track testing with a swarm of robot-driven platforms.
A single scenario with one AV under test could require several platforms like the GST and LaunchPad for close-in manoeuvres, with a further fleet of real vehicles as additional background traffic actors that are either robot or human-driven. This scenario may need to be replayed several times throughout the day to get consistent and valid confirmation that the test has been passed. The scenario may be further complicated by adding other AVs into the mix when testing Vehicle-to-Everything (V2X) technology. There may be tens or hundreds of scenarios to test, so it likely that duplicate AVs are tested simultaneously on segregated parts of the track by different teams.
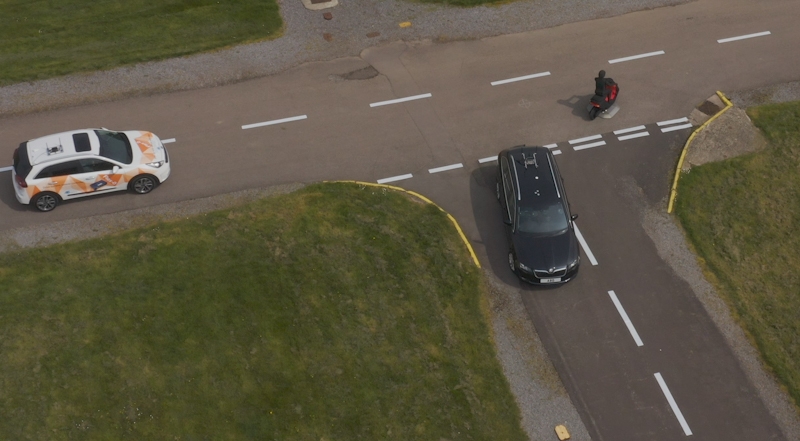
How to manage this complex new challenge
So how do we cope with this complex new world of track testing? Given the requirements for track-testing autonomous vehicles, our software and hardware solution for safety, logistics, and management of platforms must also evolve to cope with these new challenges.
With our Ground Traffic Control (GTC) system, however, we have not just made an evolutionary step, but a revolutionary step. GTC has been designed from the ground up, for the future of robot track testing, such as for the autonomous vehicle testing scenario described above. GTC is a real-time test management and monitoring solution with many features to facilitate this new complex testing regime.
GTC uses a hub-and-spoke model where the real-time GTC Server will automatically connect to all robot-controlled test vehicles including the VUT. The GTC server continuously monitors the robot systems throughout the day. Any operator at a base station can monitor, command, and control vehicles using the GTC Software. The Windows-based GTC Software provides a smooth and intuitive interface with asset management, live platform state, and a map view that provides a smooth and continuous map from Microsoft Bing.
With so many robot-driven platforms on the track, you might ask, “Surely there are going to be more accidents?”
Well, with GTC, we have solved this problem by including an advanced collision detection and prevention algorithm that will monitor the trajectory of each vehicle to ensure they can pass near each other safely without colliding. The system will allow AB Dynamics’ driving robots and platforms to gently brake to yield, merge and queue along their paths. This innovation will dramatically reduce the risk of vehicle accidents on the test track. It does not require any extra sensor technology such as radar or lidar because we already know the accurate positions using accurate and reliable GPS based navigation systems.
This will help prevent collisions between vehicles, but how can we possibly manage so many objects on the track with a small team? In our experience, a single base station operator could only monitor and manage three to four robot platforms at a time, so how could we possibly manage a large test with 16 platforms?
The GTC system will allow multiple base station operators to connect-in and see the whole picture on the track. They can monitor all vehicle movements or create testing groups where they take control of any number of vehicles to start and stop their tests. This allows multiple, but separate tests to happen on the track whilst giving situational awareness to each operator. For large testing groups, multiple base station operators can team-up and control a more substantial robot testing fleet by sharing the workload.
GTC is not just a boost to autonomous vehicle testing however, it will also revolutionise the way that other track testing activities are performed. GTC can equally increase productivity and safety of other activities such as durability and fatigue testing, dynamics testing and general proving ground management.
Get in touch with AB Dynamics to explore how your track testing could be revolutionised by GTC.
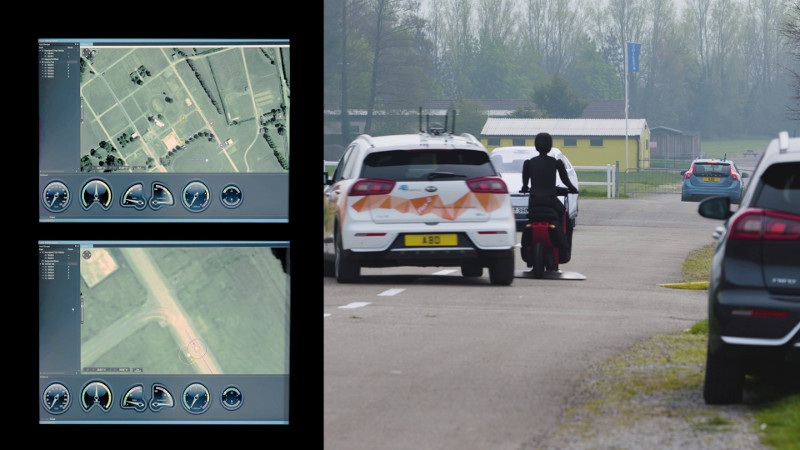